Areti Manataki
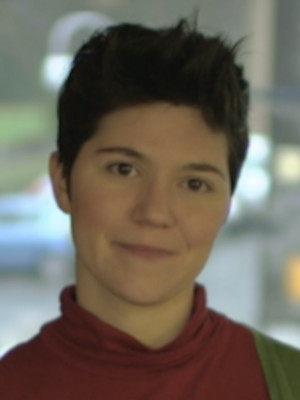
Dr Areti Manataki
Dr Areti Manataki is a Lecturer in Computer Science at the University of St Andrews. In her research, she employs artificial intelligence methods to improve the delivery of healthcare services. Her focus is on data-driven optimisation of patient flow and improvement of care pathways for patients with long-term conditions. This includes mining surgical patient flow patterns with the use of machine learning, analysing electronic health record workflow with the use of process mining, and modelling HIV care pathways with the use of formal methods.
Areti has recently developed interests in advanced data visualisation, in particular in the healthcare domain. Given the poor health literacy of the general population and the complexity of medical data, Areti thinks that there is a great opportunity for dataviz to help patients, populations and clinicians to better understand and ultimately improve their health and care.
Areti has extensive experience in leading a range of innovative health data science and computing courses, teaching undergraduate, postgraduate and professional audiences how to capitalise on the power of data to enhance human health. This includes the hugely successful Code Yourself! and ¡A Programar! courses on Coursera, which have attracted over 500,000 learners worldwide.
Prior to joining the University of St Andrews, Areti worked as a Teaching and Research Fellow and as a Senior Researcher at the University of Edinburgh, where she also obtained her PhD.
Recent Publications
- Petrov, D, Nguyen, T, Manataki, A & McCowan, C 2025, Analysing disease trajectories of multimorbidity through process mining techniques: a case study. in A Delgado & T Slaats (eds), Process mining workshops: ICPM 2024 international workshops, Lyngby, Denmark, October 14–18, 2024, revised selected papers. Lecture notes in business information processing, vol. 533, Springer Nature Switzerland AG, Cham, pp. 460-472, 6th International Conference on Process Mining, Lyngby, Denmark, 14/10/24. https://doi.org/10.1007/978-3-031-82225-4_34
- Bach, B, Keck, M, Rajabiyazdi, F, Losev, T, Meirelles, I, Dykes, J, Laramee, RS, AlKadi, M, Stoiber, C, Huron, S, Perin, C, Morais, L, Aigner, W, Kosminsky, D, Boucher, M, Knudsen, S, Manataki, A, Aerts, J, Hinrichs, U, Roberts, JC & Carpendale, S 2024, 'Challenges and opportunities in data visualization education: A call to action', IEEE Transactions on Visualization and Computer Graphics, vol. 30, no. 1, pp. 649-660. https://doi.org/10.1109/TVCG.2023.3327378
- Rohani, N, Rohani, B & Manataki, A 2024, 'ClickTree: a tree-based method for predicting math students’ performance based on clickstream data', Journal of Educational Data Mining, vol. 16, no. 2, pp. 32-57. https://doi.org/10.5281/zenodo.13627655
- Rohani, N, Gallagher, M, Gal, K & Manataki, A 2024, 'Education challenges among early career researchers in medical informatics', Studies in Health Technology and Informatics, vol. 316, pp. 1510-1514. https://doi.org/10.3233/SHTI240701
- Palmer, J, Manataki, A, Moss, L, Neilson, A & Lo, T-YM 2024, 'Feasibility of forecasting future critical care bed availability using bed management data', BMJ Health & Care Informatics, vol. 31, no. 1, e101096. https://doi.org/10.1136/bmjhci-2024-101096
- Rohani, N, Sowa, S & Manataki, A 2024, 'Identifying learning preferences and strategies in health data science courses: Systematic review', JMIR Medical Education, vol. 10, e50667. https://doi.org/10.2196/50667, https://doi.org/10.2196/50667
- Rohani, N, Gal, K, Gallagher, M & Manataki, A 2024, 'Providing insights into health data science education through artificial intelligence', BMC Medical Education, vol. 24, no. 1, 564. https://doi.org/10.1186/s12909-024-05555-3
- Rohani, N, Gallagher, M, Gal, K, Banas, K & Manataki, A 2024, 'Self-reported learning strategies and preferences in health informatics', Studies in Health Technology and Informatics, vol. 316, pp. 1540-1544. https://doi.org/10.3233/SHTI240710
- Kirkwood, JR, Dickson, J, Stevens, M, Manataki, A, Lindsay, RS, Wake, DJ & Reynolds, RM 2024, 'The user-centered design of a clinical dashboard and patient-facing app for gestational diabetes', Journal of Diabetes Science and Technology, vol. OnlineFirst. https://doi.org/10.1177/19322968241301792